Pulling the principal components out of a DimensionReducerFunction?How can I determine the importance of variables from Classify?Why is the classify function not giving the desired output?How to use Mathematica to train a network Using out of core classification?How to train a net for recognize the numberHow to train a network using out of core training when data have different length?FeatureSpacePlot freaks out with LatentSemanticAnalysis on wordsHow can I reproduce the result of DimensionReduction?How can I see the predictor function that Mathematica produces?Machine learning: How to fix part of the weight matrix?Ordinal subset in the set of classes
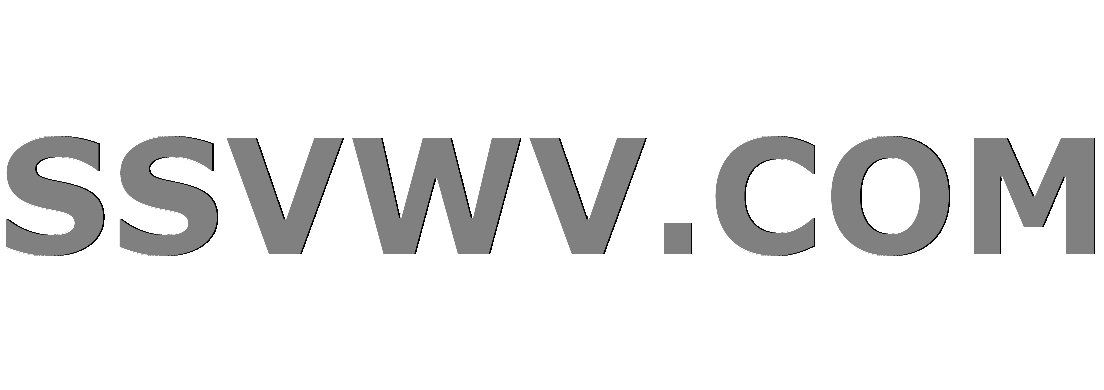
Multi tool use
Does Dispel Magic work on Tiny Hut?
Is it possible to create a QR code using text?
Machine learning testing data
What does the same-ish mean?
Solving an equation with constraints
My ex-girlfriend uses my Apple ID to log in to her iPad. Do I have to give her my Apple ID password to reset it?
Processor speed limited at 0.4 Ghz
Am I breaking OOP practice with this architecture?
How can I deal with my CEO asking me to hire someone with a higher salary than me, a co-founder?
Can I hook these wires up to find the connection to a dead outlet?
How can saying a song's name be a copyright violation?
What is the fastest integer factorization to break RSA?
How could indestructible materials be used in power generation?
Getting extremely large arrows with tikzcd
Finitely generated matrix groups whose eigenvalues are all algebraic
How to Prove P(a) → ∀x(P(x) ∨ ¬(x = a)) using Natural Deduction
What is the opposite of "eschatology"?
Ambiguity in the definition of entropy
Is this draw by repetition?
Notepad++ delete until colon for every line with replace all
Was the Stack Exchange "Happy April Fools" page fitting with the '90's code?
Are British MPs missing the point, with these 'Indicative Votes'?
Different meanings of こわい
Using "tail" to follow a file without displaying the most recent lines
Pulling the principal components out of a DimensionReducerFunction?
How can I determine the importance of variables from Classify?Why is the classify function not giving the desired output?How to use Mathematica to train a network Using out of core classification?How to train a net for recognize the numberHow to train a network using out of core training when data have different length?FeatureSpacePlot freaks out with LatentSemanticAnalysis on wordsHow can I reproduce the result of DimensionReduction?How can I see the predictor function that Mathematica produces?Machine learning: How to fix part of the weight matrix?Ordinal subset in the set of classes
$begingroup$
Suppose I perform dimension reduction using PCA:
dr = DimensionReduction[1, 2, 3, 2, 3, 5, 3, 5, 8, 4, 5,
8.5, Method -> "PrincipalComponentsAnalysis"]
If I want to see the principal components themselves, in the original space, one thought is to use the "OriginalData" feature of the DimensionReducerFunction
, on basis vectors in the new space:
In[8]:= dr[1.0, 0.0, "OriginalData"]
dr[0.0, 1.0, "OriginalData"]
Out[8]= 1.86006, 2.9998, 4.81724
Out[9]= 3.38701, 3.01026, 5.64163
Is this a reasonable thing to do, or am I misinterpreting how the "OriginalData" feature works? And is there a better way to pull out the principal components themselves? People often want to visualize these for various reasons.
(There are several other questions about how to solve a similar problem with the PrincipalComponents
function; this is a question about a different function.)
machine-learning dimension-reduction
$endgroup$
add a comment |
$begingroup$
Suppose I perform dimension reduction using PCA:
dr = DimensionReduction[1, 2, 3, 2, 3, 5, 3, 5, 8, 4, 5,
8.5, Method -> "PrincipalComponentsAnalysis"]
If I want to see the principal components themselves, in the original space, one thought is to use the "OriginalData" feature of the DimensionReducerFunction
, on basis vectors in the new space:
In[8]:= dr[1.0, 0.0, "OriginalData"]
dr[0.0, 1.0, "OriginalData"]
Out[8]= 1.86006, 2.9998, 4.81724
Out[9]= 3.38701, 3.01026, 5.64163
Is this a reasonable thing to do, or am I misinterpreting how the "OriginalData" feature works? And is there a better way to pull out the principal components themselves? People often want to visualize these for various reasons.
(There are several other questions about how to solve a similar problem with the PrincipalComponents
function; this is a question about a different function.)
machine-learning dimension-reduction
$endgroup$
add a comment |
$begingroup$
Suppose I perform dimension reduction using PCA:
dr = DimensionReduction[1, 2, 3, 2, 3, 5, 3, 5, 8, 4, 5,
8.5, Method -> "PrincipalComponentsAnalysis"]
If I want to see the principal components themselves, in the original space, one thought is to use the "OriginalData" feature of the DimensionReducerFunction
, on basis vectors in the new space:
In[8]:= dr[1.0, 0.0, "OriginalData"]
dr[0.0, 1.0, "OriginalData"]
Out[8]= 1.86006, 2.9998, 4.81724
Out[9]= 3.38701, 3.01026, 5.64163
Is this a reasonable thing to do, or am I misinterpreting how the "OriginalData" feature works? And is there a better way to pull out the principal components themselves? People often want to visualize these for various reasons.
(There are several other questions about how to solve a similar problem with the PrincipalComponents
function; this is a question about a different function.)
machine-learning dimension-reduction
$endgroup$
Suppose I perform dimension reduction using PCA:
dr = DimensionReduction[1, 2, 3, 2, 3, 5, 3, 5, 8, 4, 5,
8.5, Method -> "PrincipalComponentsAnalysis"]
If I want to see the principal components themselves, in the original space, one thought is to use the "OriginalData" feature of the DimensionReducerFunction
, on basis vectors in the new space:
In[8]:= dr[1.0, 0.0, "OriginalData"]
dr[0.0, 1.0, "OriginalData"]
Out[8]= 1.86006, 2.9998, 4.81724
Out[9]= 3.38701, 3.01026, 5.64163
Is this a reasonable thing to do, or am I misinterpreting how the "OriginalData" feature works? And is there a better way to pull out the principal components themselves? People often want to visualize these for various reasons.
(There are several other questions about how to solve a similar problem with the PrincipalComponents
function; this is a question about a different function.)
machine-learning dimension-reduction
machine-learning dimension-reduction
edited 2 days ago
J. M. is slightly pensive♦
98.9k10311467
98.9k10311467
asked 2 days ago
Michael CurryMichael Curry
786312
786312
add a comment |
add a comment |
1 Answer
1
active
oldest
votes
$begingroup$
Here's your data:
data = 1, 2, 3, 2, 3, 5, 3, 5, 8, 4, 5, 8.5;
dr = DimensionReduction[data, Method -> "PrincipalComponentsAnalysis"];
This is not exactly a top level solution, but we can pick apart the DimensionReducerFunction
and see inside (try dr[[1]]
to see many internal properties).
Looking further, in there we have a matrix:
Transpose[dr[[1, "Model", "Matrix"]]]
-0.572383, -0.577502, -0.582125, 0.793367, -0.56945, -0.215163
I think these are the components. We can try to verify:
Transpose[Last[SingularValueDecomposition[Standardize[data], 2]]]
-0.572383, -0.577502, -0.582125, 0.793367, -0.56945, -0.215163
$endgroup$
2
$begingroup$
It doesn't look like you need the pre-multiplication by2/Sqrt[3]
; after all, any such rescaling would show itself in the singular values and not the orthogonal factors. The important thing is the shift, whichStandardize[]
of course does.
$endgroup$
– J. M. is slightly pensive♦
2 days ago
$begingroup$
Good catch, I've edited the post. I can't remember what I ran into that led me to such a conclusion.
$endgroup$
– Chip Hurst
2 days ago
add a comment |
Your Answer
StackExchange.ifUsing("editor", function ()
return StackExchange.using("mathjaxEditing", function ()
StackExchange.MarkdownEditor.creationCallbacks.add(function (editor, postfix)
StackExchange.mathjaxEditing.prepareWmdForMathJax(editor, postfix, [["$", "$"], ["\\(","\\)"]]);
);
);
, "mathjax-editing");
StackExchange.ready(function()
var channelOptions =
tags: "".split(" "),
id: "387"
;
initTagRenderer("".split(" "), "".split(" "), channelOptions);
StackExchange.using("externalEditor", function()
// Have to fire editor after snippets, if snippets enabled
if (StackExchange.settings.snippets.snippetsEnabled)
StackExchange.using("snippets", function()
createEditor();
);
else
createEditor();
);
function createEditor()
StackExchange.prepareEditor(
heartbeatType: 'answer',
autoActivateHeartbeat: false,
convertImagesToLinks: false,
noModals: true,
showLowRepImageUploadWarning: true,
reputationToPostImages: null,
bindNavPrevention: true,
postfix: "",
imageUploader:
brandingHtml: "Powered by u003ca class="icon-imgur-white" href="https://imgur.com/"u003eu003c/au003e",
contentPolicyHtml: "User contributions licensed under u003ca href="https://creativecommons.org/licenses/by-sa/3.0/"u003ecc by-sa 3.0 with attribution requiredu003c/au003e u003ca href="https://stackoverflow.com/legal/content-policy"u003e(content policy)u003c/au003e",
allowUrls: true
,
onDemand: true,
discardSelector: ".discard-answer"
,immediatelyShowMarkdownHelp:true
);
);
Sign up or log in
StackExchange.ready(function ()
StackExchange.helpers.onClickDraftSave('#login-link');
);
Sign up using Google
Sign up using Facebook
Sign up using Email and Password
Post as a guest
Required, but never shown
StackExchange.ready(
function ()
StackExchange.openid.initPostLogin('.new-post-login', 'https%3a%2f%2fmathematica.stackexchange.com%2fquestions%2f194324%2fpulling-the-principal-components-out-of-a-dimensionreducerfunction%23new-answer', 'question_page');
);
Post as a guest
Required, but never shown
1 Answer
1
active
oldest
votes
1 Answer
1
active
oldest
votes
active
oldest
votes
active
oldest
votes
$begingroup$
Here's your data:
data = 1, 2, 3, 2, 3, 5, 3, 5, 8, 4, 5, 8.5;
dr = DimensionReduction[data, Method -> "PrincipalComponentsAnalysis"];
This is not exactly a top level solution, but we can pick apart the DimensionReducerFunction
and see inside (try dr[[1]]
to see many internal properties).
Looking further, in there we have a matrix:
Transpose[dr[[1, "Model", "Matrix"]]]
-0.572383, -0.577502, -0.582125, 0.793367, -0.56945, -0.215163
I think these are the components. We can try to verify:
Transpose[Last[SingularValueDecomposition[Standardize[data], 2]]]
-0.572383, -0.577502, -0.582125, 0.793367, -0.56945, -0.215163
$endgroup$
2
$begingroup$
It doesn't look like you need the pre-multiplication by2/Sqrt[3]
; after all, any such rescaling would show itself in the singular values and not the orthogonal factors. The important thing is the shift, whichStandardize[]
of course does.
$endgroup$
– J. M. is slightly pensive♦
2 days ago
$begingroup$
Good catch, I've edited the post. I can't remember what I ran into that led me to such a conclusion.
$endgroup$
– Chip Hurst
2 days ago
add a comment |
$begingroup$
Here's your data:
data = 1, 2, 3, 2, 3, 5, 3, 5, 8, 4, 5, 8.5;
dr = DimensionReduction[data, Method -> "PrincipalComponentsAnalysis"];
This is not exactly a top level solution, but we can pick apart the DimensionReducerFunction
and see inside (try dr[[1]]
to see many internal properties).
Looking further, in there we have a matrix:
Transpose[dr[[1, "Model", "Matrix"]]]
-0.572383, -0.577502, -0.582125, 0.793367, -0.56945, -0.215163
I think these are the components. We can try to verify:
Transpose[Last[SingularValueDecomposition[Standardize[data], 2]]]
-0.572383, -0.577502, -0.582125, 0.793367, -0.56945, -0.215163
$endgroup$
2
$begingroup$
It doesn't look like you need the pre-multiplication by2/Sqrt[3]
; after all, any such rescaling would show itself in the singular values and not the orthogonal factors. The important thing is the shift, whichStandardize[]
of course does.
$endgroup$
– J. M. is slightly pensive♦
2 days ago
$begingroup$
Good catch, I've edited the post. I can't remember what I ran into that led me to such a conclusion.
$endgroup$
– Chip Hurst
2 days ago
add a comment |
$begingroup$
Here's your data:
data = 1, 2, 3, 2, 3, 5, 3, 5, 8, 4, 5, 8.5;
dr = DimensionReduction[data, Method -> "PrincipalComponentsAnalysis"];
This is not exactly a top level solution, but we can pick apart the DimensionReducerFunction
and see inside (try dr[[1]]
to see many internal properties).
Looking further, in there we have a matrix:
Transpose[dr[[1, "Model", "Matrix"]]]
-0.572383, -0.577502, -0.582125, 0.793367, -0.56945, -0.215163
I think these are the components. We can try to verify:
Transpose[Last[SingularValueDecomposition[Standardize[data], 2]]]
-0.572383, -0.577502, -0.582125, 0.793367, -0.56945, -0.215163
$endgroup$
Here's your data:
data = 1, 2, 3, 2, 3, 5, 3, 5, 8, 4, 5, 8.5;
dr = DimensionReduction[data, Method -> "PrincipalComponentsAnalysis"];
This is not exactly a top level solution, but we can pick apart the DimensionReducerFunction
and see inside (try dr[[1]]
to see many internal properties).
Looking further, in there we have a matrix:
Transpose[dr[[1, "Model", "Matrix"]]]
-0.572383, -0.577502, -0.582125, 0.793367, -0.56945, -0.215163
I think these are the components. We can try to verify:
Transpose[Last[SingularValueDecomposition[Standardize[data], 2]]]
-0.572383, -0.577502, -0.582125, 0.793367, -0.56945, -0.215163
edited 2 days ago
answered 2 days ago


Chip HurstChip Hurst
23k15893
23k15893
2
$begingroup$
It doesn't look like you need the pre-multiplication by2/Sqrt[3]
; after all, any such rescaling would show itself in the singular values and not the orthogonal factors. The important thing is the shift, whichStandardize[]
of course does.
$endgroup$
– J. M. is slightly pensive♦
2 days ago
$begingroup$
Good catch, I've edited the post. I can't remember what I ran into that led me to such a conclusion.
$endgroup$
– Chip Hurst
2 days ago
add a comment |
2
$begingroup$
It doesn't look like you need the pre-multiplication by2/Sqrt[3]
; after all, any such rescaling would show itself in the singular values and not the orthogonal factors. The important thing is the shift, whichStandardize[]
of course does.
$endgroup$
– J. M. is slightly pensive♦
2 days ago
$begingroup$
Good catch, I've edited the post. I can't remember what I ran into that led me to such a conclusion.
$endgroup$
– Chip Hurst
2 days ago
2
2
$begingroup$
It doesn't look like you need the pre-multiplication by
2/Sqrt[3]
; after all, any such rescaling would show itself in the singular values and not the orthogonal factors. The important thing is the shift, which Standardize[]
of course does.$endgroup$
– J. M. is slightly pensive♦
2 days ago
$begingroup$
It doesn't look like you need the pre-multiplication by
2/Sqrt[3]
; after all, any such rescaling would show itself in the singular values and not the orthogonal factors. The important thing is the shift, which Standardize[]
of course does.$endgroup$
– J. M. is slightly pensive♦
2 days ago
$begingroup$
Good catch, I've edited the post. I can't remember what I ran into that led me to such a conclusion.
$endgroup$
– Chip Hurst
2 days ago
$begingroup$
Good catch, I've edited the post. I can't remember what I ran into that led me to such a conclusion.
$endgroup$
– Chip Hurst
2 days ago
add a comment |
Thanks for contributing an answer to Mathematica Stack Exchange!
- Please be sure to answer the question. Provide details and share your research!
But avoid …
- Asking for help, clarification, or responding to other answers.
- Making statements based on opinion; back them up with references or personal experience.
Use MathJax to format equations. MathJax reference.
To learn more, see our tips on writing great answers.
Sign up or log in
StackExchange.ready(function ()
StackExchange.helpers.onClickDraftSave('#login-link');
);
Sign up using Google
Sign up using Facebook
Sign up using Email and Password
Post as a guest
Required, but never shown
StackExchange.ready(
function ()
StackExchange.openid.initPostLogin('.new-post-login', 'https%3a%2f%2fmathematica.stackexchange.com%2fquestions%2f194324%2fpulling-the-principal-components-out-of-a-dimensionreducerfunction%23new-answer', 'question_page');
);
Post as a guest
Required, but never shown
Sign up or log in
StackExchange.ready(function ()
StackExchange.helpers.onClickDraftSave('#login-link');
);
Sign up using Google
Sign up using Facebook
Sign up using Email and Password
Post as a guest
Required, but never shown
Sign up or log in
StackExchange.ready(function ()
StackExchange.helpers.onClickDraftSave('#login-link');
);
Sign up using Google
Sign up using Facebook
Sign up using Email and Password
Post as a guest
Required, but never shown
Sign up or log in
StackExchange.ready(function ()
StackExchange.helpers.onClickDraftSave('#login-link');
);
Sign up using Google
Sign up using Facebook
Sign up using Email and Password
Sign up using Google
Sign up using Facebook
Sign up using Email and Password
Post as a guest
Required, but never shown
Required, but never shown
Required, but never shown
Required, but never shown
Required, but never shown
Required, but never shown
Required, but never shown
Required, but never shown
Required, but never shown
Pynn1YyUaEU3cLsZvMpH6zehgAGRIxzKJcN6YfzZysTt2v,OtOjE mfDa0o5cxGwITVc038A,vjs0ZK aTbdkM7LE4WKNOsi