Creating thinned models in during Dropout processMathematically modeling neural networks as graphical modelsQuestions about understanding convolutional neural network (with Tensorflow's example)How to efficiently and un-biasedly evaluate a deep net for classification taskRecursive neural networks for Part-of-speech tagging?Why do Srivastava et al. claim that “the best” theoretical regularization technique involves all possible network parameter settings?Understanding dropout method: one bask per batch, or more?Why is dropout causing my network to overfit so badly?Dropout in Deep Neural NetworksNeural Networks Mappings( Topology)What does it mean by “approach the performance of the Bayesian gold standard”?
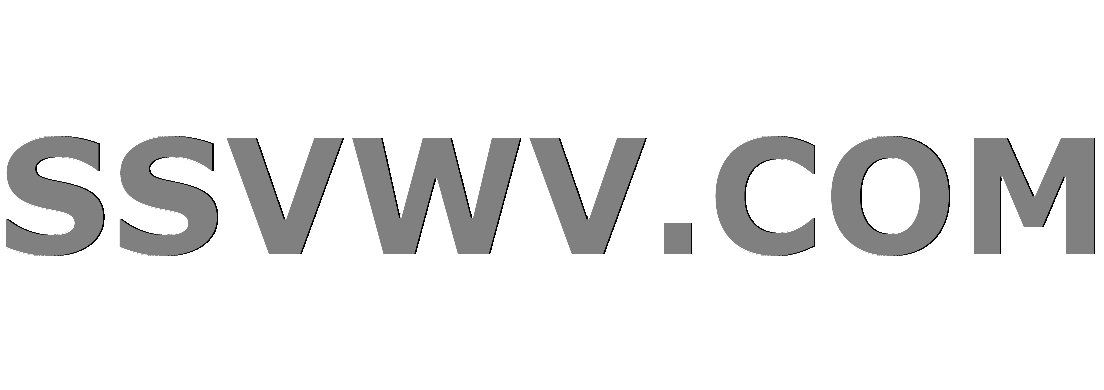
Multi tool use
Is this Pascal's Matrix?
Could any one tell what PN is this Chip? Thanks~
How to test the sharpness of a knife?
Writing in a Christian voice
Why do I have a large white artefact on the rendered image?
What will the Frenchman say?
"Marked down as someone wanting to sell shares." What does that mean?
Single word to change groups
Homology of the fiber
pipe commands inside find -exec?
What is it called when someone votes for an option that's not their first choice?
Can "few" be used as a subject? If so, what is the rule?
is this saw blade faulty?
What (if any) is the reason to buy in small local stores?
Does fire aspect on a sword, destroy mob drops?
Help with identifying unique aircraft over NE Pennsylvania
Are hand made posters acceptable in Academia?
Is VPN a layer 3 concept?
Symbolism of 18 Journeyers
Gauss brackets with double vertical lines
Why is this tree refusing to shed its dead leaves?
Justification failure in beamer enumerate list
TDE Master Key Rotation
Can other pieces capture a threatening piece and prevent a checkmate?
Creating thinned models in during Dropout process
Mathematically modeling neural networks as graphical modelsQuestions about understanding convolutional neural network (with Tensorflow's example)How to efficiently and un-biasedly evaluate a deep net for classification taskRecursive neural networks for Part-of-speech tagging?Why do Srivastava et al. claim that “the best” theoretical regularization technique involves all possible network parameter settings?Understanding dropout method: one bask per batch, or more?Why is dropout causing my network to overfit so badly?Dropout in Deep Neural NetworksNeural Networks Mappings( Topology)What does it mean by “approach the performance of the Bayesian gold standard”?
$begingroup$
Applying dropout to a neural network amounts to sampling a “thinned” network from it. The thinned network consists of all the units that survived dropout. A neural net with n units can be seen as a collection of 2^n possible thinned neural networks.
Source:
Dropout: A Simple Way to Prevent Neural Networks fromOverfitting, pg. 1931.
How are we getting these 2^n models?
machine-learning deep-learning dropout
New contributor
ashirwad is a new contributor to this site. Take care in asking for clarification, commenting, and answering.
Check out our Code of Conduct.
$endgroup$
add a comment |
$begingroup$
Applying dropout to a neural network amounts to sampling a “thinned” network from it. The thinned network consists of all the units that survived dropout. A neural net with n units can be seen as a collection of 2^n possible thinned neural networks.
Source:
Dropout: A Simple Way to Prevent Neural Networks fromOverfitting, pg. 1931.
How are we getting these 2^n models?
machine-learning deep-learning dropout
New contributor
ashirwad is a new contributor to this site. Take care in asking for clarification, commenting, and answering.
Check out our Code of Conduct.
$endgroup$
add a comment |
$begingroup$
Applying dropout to a neural network amounts to sampling a “thinned” network from it. The thinned network consists of all the units that survived dropout. A neural net with n units can be seen as a collection of 2^n possible thinned neural networks.
Source:
Dropout: A Simple Way to Prevent Neural Networks fromOverfitting, pg. 1931.
How are we getting these 2^n models?
machine-learning deep-learning dropout
New contributor
ashirwad is a new contributor to this site. Take care in asking for clarification, commenting, and answering.
Check out our Code of Conduct.
$endgroup$
Applying dropout to a neural network amounts to sampling a “thinned” network from it. The thinned network consists of all the units that survived dropout. A neural net with n units can be seen as a collection of 2^n possible thinned neural networks.
Source:
Dropout: A Simple Way to Prevent Neural Networks fromOverfitting, pg. 1931.
How are we getting these 2^n models?
machine-learning deep-learning dropout
machine-learning deep-learning dropout
New contributor
ashirwad is a new contributor to this site. Take care in asking for clarification, commenting, and answering.
Check out our Code of Conduct.
New contributor
ashirwad is a new contributor to this site. Take care in asking for clarification, commenting, and answering.
Check out our Code of Conduct.
edited 14 hours ago
Djib2011
2,58931125
2,58931125
New contributor
ashirwad is a new contributor to this site. Take care in asking for clarification, commenting, and answering.
Check out our Code of Conduct.
asked 15 hours ago


ashirwadashirwad
213
213
New contributor
ashirwad is a new contributor to this site. Take care in asking for clarification, commenting, and answering.
Check out our Code of Conduct.
New contributor
ashirwad is a new contributor to this site. Take care in asking for clarification, commenting, and answering.
Check out our Code of Conduct.
ashirwad is a new contributor to this site. Take care in asking for clarification, commenting, and answering.
Check out our Code of Conduct.
add a comment |
add a comment |
2 Answers
2
active
oldest
votes
$begingroup$
The statement is a bit oversimplifying but the idea is that assuming we have $n$ nodes and each of these nodes might be "dropped", we have $2^n$ possible thinned neural networks. Obviously dropping out an entire layer would alter the whole structure of the network but the idea is straightforward: we ignore the activation/information from certain randomly selected neurons and thus encourage redundancy learning and discourage over-fitting on very specific features.
The same idea has also been employed in Gradient Boosting Machines where instead of "ignoring neurons" we "ignore trees" at random (see Rashmi & Gilad-Bachrach (2015) DART: Dropouts meet Multiple Additive Regression Trees on that matter).
Minor edit: I just saw Djib2011's answer. (+1) He/she specifically shows why the statement is somewhat over-simplifying. If we assume that we can drop any (or all, or none) of the neurons we have $2^n$ possible networks.
$endgroup$
add a comment |
$begingroup$
I too haven't understood their reasoning, I always assumed it was a typo or something...
The way I see it we if we have $n$ hidden units in a Neural Network with a single hidden layer and we apply dropout keeping $r$ of those, we'll have:
$$
fracn!r! cdot (n-r)!
$$
possible combinations (not $2^n$ as the authors state).
Example:
Assume a simple fully connected neural network with a single hidden layer with 4 neurons. This means the hidden layer will have 4 outputs $h_1, h_2, h_3, h_4$.
Now, you want to apply dropout to this layer with a 0.5 probability (i.e. half of the outputs will be dropped).
Since 2 out of the 4 outputs will be dropped, at each training iteration we'll have one of the following possibilities:
- $h_1, h_2$
- $h_1, h_3$
- $h_1, h_4$
- $h_2, h_3$
- $h_2, h_4$
- $h_3, h_4$
or by applying the formula:
$$
frac4!2! cdot (4-2)! = frac242 cdot 2 = 6
$$
$endgroup$
3
$begingroup$
I do not think that most implementations of dropout work by saying: If there are 100 neurons, and the probability is 0.05, I have to disable exactly 5 neurons chosen at random. Instead each neuron is disabled with a probability of 0.05, independently of what happens with the rest. Hence, the cases where all or no neurons are disabled, while unlikely, are possible.
$endgroup$
– Daniel López
13 hours ago
1
$begingroup$
@DanielLópez: I think both you and Djib2011 (+1 both) are "factually correct" on this. The statement is clearly oversimplifying things. You also need to take into account that most the networks that this paper is concerned with, have thousands of neurons so certain it is kind of accepted that no layer will be totally switched off.
$endgroup$
– usεr11852
13 hours ago
2
$begingroup$
Agree, but I believe the above example is transmitting the idea that exactly $n cdot textprob$ units are disabled with dropout, where $textprob$ is the dropout probability. And this is not how dropout works.
$endgroup$
– Daniel López
13 hours ago
$begingroup$
Well... LLN is our friend. :)
$endgroup$
– usεr11852
13 hours ago
$begingroup$
The flaw with the reasoning presented here is that dropout sets weights to 0 with some fixed probability independently. This implies that the number of zero weights at each step has a binomial distribution, because dropout has the three defining characteristics of a binomial distribution 1 dichotomous outcomes (weights are on or off) 2 fixed number of trials (number of weights in the model doesn't change) 3 probability of success is fixed & independent for each trial.
$endgroup$
– Sycorax
1 hour ago
add a comment |
Your Answer
StackExchange.ifUsing("editor", function ()
return StackExchange.using("mathjaxEditing", function ()
StackExchange.MarkdownEditor.creationCallbacks.add(function (editor, postfix)
StackExchange.mathjaxEditing.prepareWmdForMathJax(editor, postfix, [["$", "$"], ["\\(","\\)"]]);
);
);
, "mathjax-editing");
StackExchange.ready(function()
var channelOptions =
tags: "".split(" "),
id: "65"
;
initTagRenderer("".split(" "), "".split(" "), channelOptions);
StackExchange.using("externalEditor", function()
// Have to fire editor after snippets, if snippets enabled
if (StackExchange.settings.snippets.snippetsEnabled)
StackExchange.using("snippets", function()
createEditor();
);
else
createEditor();
);
function createEditor()
StackExchange.prepareEditor(
heartbeatType: 'answer',
autoActivateHeartbeat: false,
convertImagesToLinks: false,
noModals: true,
showLowRepImageUploadWarning: true,
reputationToPostImages: null,
bindNavPrevention: true,
postfix: "",
imageUploader:
brandingHtml: "Powered by u003ca class="icon-imgur-white" href="https://imgur.com/"u003eu003c/au003e",
contentPolicyHtml: "User contributions licensed under u003ca href="https://creativecommons.org/licenses/by-sa/3.0/"u003ecc by-sa 3.0 with attribution requiredu003c/au003e u003ca href="https://stackoverflow.com/legal/content-policy"u003e(content policy)u003c/au003e",
allowUrls: true
,
onDemand: true,
discardSelector: ".discard-answer"
,immediatelyShowMarkdownHelp:true
);
);
ashirwad is a new contributor. Be nice, and check out our Code of Conduct.
Sign up or log in
StackExchange.ready(function ()
StackExchange.helpers.onClickDraftSave('#login-link');
);
Sign up using Google
Sign up using Facebook
Sign up using Email and Password
Post as a guest
Required, but never shown
StackExchange.ready(
function ()
StackExchange.openid.initPostLogin('.new-post-login', 'https%3a%2f%2fstats.stackexchange.com%2fquestions%2f398114%2fcreating-thinned-models-in-during-dropout-process%23new-answer', 'question_page');
);
Post as a guest
Required, but never shown
2 Answers
2
active
oldest
votes
2 Answers
2
active
oldest
votes
active
oldest
votes
active
oldest
votes
$begingroup$
The statement is a bit oversimplifying but the idea is that assuming we have $n$ nodes and each of these nodes might be "dropped", we have $2^n$ possible thinned neural networks. Obviously dropping out an entire layer would alter the whole structure of the network but the idea is straightforward: we ignore the activation/information from certain randomly selected neurons and thus encourage redundancy learning and discourage over-fitting on very specific features.
The same idea has also been employed in Gradient Boosting Machines where instead of "ignoring neurons" we "ignore trees" at random (see Rashmi & Gilad-Bachrach (2015) DART: Dropouts meet Multiple Additive Regression Trees on that matter).
Minor edit: I just saw Djib2011's answer. (+1) He/she specifically shows why the statement is somewhat over-simplifying. If we assume that we can drop any (or all, or none) of the neurons we have $2^n$ possible networks.
$endgroup$
add a comment |
$begingroup$
The statement is a bit oversimplifying but the idea is that assuming we have $n$ nodes and each of these nodes might be "dropped", we have $2^n$ possible thinned neural networks. Obviously dropping out an entire layer would alter the whole structure of the network but the idea is straightforward: we ignore the activation/information from certain randomly selected neurons and thus encourage redundancy learning and discourage over-fitting on very specific features.
The same idea has also been employed in Gradient Boosting Machines where instead of "ignoring neurons" we "ignore trees" at random (see Rashmi & Gilad-Bachrach (2015) DART: Dropouts meet Multiple Additive Regression Trees on that matter).
Minor edit: I just saw Djib2011's answer. (+1) He/she specifically shows why the statement is somewhat over-simplifying. If we assume that we can drop any (or all, or none) of the neurons we have $2^n$ possible networks.
$endgroup$
add a comment |
$begingroup$
The statement is a bit oversimplifying but the idea is that assuming we have $n$ nodes and each of these nodes might be "dropped", we have $2^n$ possible thinned neural networks. Obviously dropping out an entire layer would alter the whole structure of the network but the idea is straightforward: we ignore the activation/information from certain randomly selected neurons and thus encourage redundancy learning and discourage over-fitting on very specific features.
The same idea has also been employed in Gradient Boosting Machines where instead of "ignoring neurons" we "ignore trees" at random (see Rashmi & Gilad-Bachrach (2015) DART: Dropouts meet Multiple Additive Regression Trees on that matter).
Minor edit: I just saw Djib2011's answer. (+1) He/she specifically shows why the statement is somewhat over-simplifying. If we assume that we can drop any (or all, or none) of the neurons we have $2^n$ possible networks.
$endgroup$
The statement is a bit oversimplifying but the idea is that assuming we have $n$ nodes and each of these nodes might be "dropped", we have $2^n$ possible thinned neural networks. Obviously dropping out an entire layer would alter the whole structure of the network but the idea is straightforward: we ignore the activation/information from certain randomly selected neurons and thus encourage redundancy learning and discourage over-fitting on very specific features.
The same idea has also been employed in Gradient Boosting Machines where instead of "ignoring neurons" we "ignore trees" at random (see Rashmi & Gilad-Bachrach (2015) DART: Dropouts meet Multiple Additive Regression Trees on that matter).
Minor edit: I just saw Djib2011's answer. (+1) He/she specifically shows why the statement is somewhat over-simplifying. If we assume that we can drop any (or all, or none) of the neurons we have $2^n$ possible networks.
edited 13 hours ago
answered 13 hours ago


usεr11852usεr11852
19.3k14274
19.3k14274
add a comment |
add a comment |
$begingroup$
I too haven't understood their reasoning, I always assumed it was a typo or something...
The way I see it we if we have $n$ hidden units in a Neural Network with a single hidden layer and we apply dropout keeping $r$ of those, we'll have:
$$
fracn!r! cdot (n-r)!
$$
possible combinations (not $2^n$ as the authors state).
Example:
Assume a simple fully connected neural network with a single hidden layer with 4 neurons. This means the hidden layer will have 4 outputs $h_1, h_2, h_3, h_4$.
Now, you want to apply dropout to this layer with a 0.5 probability (i.e. half of the outputs will be dropped).
Since 2 out of the 4 outputs will be dropped, at each training iteration we'll have one of the following possibilities:
- $h_1, h_2$
- $h_1, h_3$
- $h_1, h_4$
- $h_2, h_3$
- $h_2, h_4$
- $h_3, h_4$
or by applying the formula:
$$
frac4!2! cdot (4-2)! = frac242 cdot 2 = 6
$$
$endgroup$
3
$begingroup$
I do not think that most implementations of dropout work by saying: If there are 100 neurons, and the probability is 0.05, I have to disable exactly 5 neurons chosen at random. Instead each neuron is disabled with a probability of 0.05, independently of what happens with the rest. Hence, the cases where all or no neurons are disabled, while unlikely, are possible.
$endgroup$
– Daniel López
13 hours ago
1
$begingroup$
@DanielLópez: I think both you and Djib2011 (+1 both) are "factually correct" on this. The statement is clearly oversimplifying things. You also need to take into account that most the networks that this paper is concerned with, have thousands of neurons so certain it is kind of accepted that no layer will be totally switched off.
$endgroup$
– usεr11852
13 hours ago
2
$begingroup$
Agree, but I believe the above example is transmitting the idea that exactly $n cdot textprob$ units are disabled with dropout, where $textprob$ is the dropout probability. And this is not how dropout works.
$endgroup$
– Daniel López
13 hours ago
$begingroup$
Well... LLN is our friend. :)
$endgroup$
– usεr11852
13 hours ago
$begingroup$
The flaw with the reasoning presented here is that dropout sets weights to 0 with some fixed probability independently. This implies that the number of zero weights at each step has a binomial distribution, because dropout has the three defining characteristics of a binomial distribution 1 dichotomous outcomes (weights are on or off) 2 fixed number of trials (number of weights in the model doesn't change) 3 probability of success is fixed & independent for each trial.
$endgroup$
– Sycorax
1 hour ago
add a comment |
$begingroup$
I too haven't understood their reasoning, I always assumed it was a typo or something...
The way I see it we if we have $n$ hidden units in a Neural Network with a single hidden layer and we apply dropout keeping $r$ of those, we'll have:
$$
fracn!r! cdot (n-r)!
$$
possible combinations (not $2^n$ as the authors state).
Example:
Assume a simple fully connected neural network with a single hidden layer with 4 neurons. This means the hidden layer will have 4 outputs $h_1, h_2, h_3, h_4$.
Now, you want to apply dropout to this layer with a 0.5 probability (i.e. half of the outputs will be dropped).
Since 2 out of the 4 outputs will be dropped, at each training iteration we'll have one of the following possibilities:
- $h_1, h_2$
- $h_1, h_3$
- $h_1, h_4$
- $h_2, h_3$
- $h_2, h_4$
- $h_3, h_4$
or by applying the formula:
$$
frac4!2! cdot (4-2)! = frac242 cdot 2 = 6
$$
$endgroup$
3
$begingroup$
I do not think that most implementations of dropout work by saying: If there are 100 neurons, and the probability is 0.05, I have to disable exactly 5 neurons chosen at random. Instead each neuron is disabled with a probability of 0.05, independently of what happens with the rest. Hence, the cases where all or no neurons are disabled, while unlikely, are possible.
$endgroup$
– Daniel López
13 hours ago
1
$begingroup$
@DanielLópez: I think both you and Djib2011 (+1 both) are "factually correct" on this. The statement is clearly oversimplifying things. You also need to take into account that most the networks that this paper is concerned with, have thousands of neurons so certain it is kind of accepted that no layer will be totally switched off.
$endgroup$
– usεr11852
13 hours ago
2
$begingroup$
Agree, but I believe the above example is transmitting the idea that exactly $n cdot textprob$ units are disabled with dropout, where $textprob$ is the dropout probability. And this is not how dropout works.
$endgroup$
– Daniel López
13 hours ago
$begingroup$
Well... LLN is our friend. :)
$endgroup$
– usεr11852
13 hours ago
$begingroup$
The flaw with the reasoning presented here is that dropout sets weights to 0 with some fixed probability independently. This implies that the number of zero weights at each step has a binomial distribution, because dropout has the three defining characteristics of a binomial distribution 1 dichotomous outcomes (weights are on or off) 2 fixed number of trials (number of weights in the model doesn't change) 3 probability of success is fixed & independent for each trial.
$endgroup$
– Sycorax
1 hour ago
add a comment |
$begingroup$
I too haven't understood their reasoning, I always assumed it was a typo or something...
The way I see it we if we have $n$ hidden units in a Neural Network with a single hidden layer and we apply dropout keeping $r$ of those, we'll have:
$$
fracn!r! cdot (n-r)!
$$
possible combinations (not $2^n$ as the authors state).
Example:
Assume a simple fully connected neural network with a single hidden layer with 4 neurons. This means the hidden layer will have 4 outputs $h_1, h_2, h_3, h_4$.
Now, you want to apply dropout to this layer with a 0.5 probability (i.e. half of the outputs will be dropped).
Since 2 out of the 4 outputs will be dropped, at each training iteration we'll have one of the following possibilities:
- $h_1, h_2$
- $h_1, h_3$
- $h_1, h_4$
- $h_2, h_3$
- $h_2, h_4$
- $h_3, h_4$
or by applying the formula:
$$
frac4!2! cdot (4-2)! = frac242 cdot 2 = 6
$$
$endgroup$
I too haven't understood their reasoning, I always assumed it was a typo or something...
The way I see it we if we have $n$ hidden units in a Neural Network with a single hidden layer and we apply dropout keeping $r$ of those, we'll have:
$$
fracn!r! cdot (n-r)!
$$
possible combinations (not $2^n$ as the authors state).
Example:
Assume a simple fully connected neural network with a single hidden layer with 4 neurons. This means the hidden layer will have 4 outputs $h_1, h_2, h_3, h_4$.
Now, you want to apply dropout to this layer with a 0.5 probability (i.e. half of the outputs will be dropped).
Since 2 out of the 4 outputs will be dropped, at each training iteration we'll have one of the following possibilities:
- $h_1, h_2$
- $h_1, h_3$
- $h_1, h_4$
- $h_2, h_3$
- $h_2, h_4$
- $h_3, h_4$
or by applying the formula:
$$
frac4!2! cdot (4-2)! = frac242 cdot 2 = 6
$$
answered 13 hours ago
Djib2011Djib2011
2,58931125
2,58931125
3
$begingroup$
I do not think that most implementations of dropout work by saying: If there are 100 neurons, and the probability is 0.05, I have to disable exactly 5 neurons chosen at random. Instead each neuron is disabled with a probability of 0.05, independently of what happens with the rest. Hence, the cases where all or no neurons are disabled, while unlikely, are possible.
$endgroup$
– Daniel López
13 hours ago
1
$begingroup$
@DanielLópez: I think both you and Djib2011 (+1 both) are "factually correct" on this. The statement is clearly oversimplifying things. You also need to take into account that most the networks that this paper is concerned with, have thousands of neurons so certain it is kind of accepted that no layer will be totally switched off.
$endgroup$
– usεr11852
13 hours ago
2
$begingroup$
Agree, but I believe the above example is transmitting the idea that exactly $n cdot textprob$ units are disabled with dropout, where $textprob$ is the dropout probability. And this is not how dropout works.
$endgroup$
– Daniel López
13 hours ago
$begingroup$
Well... LLN is our friend. :)
$endgroup$
– usεr11852
13 hours ago
$begingroup$
The flaw with the reasoning presented here is that dropout sets weights to 0 with some fixed probability independently. This implies that the number of zero weights at each step has a binomial distribution, because dropout has the three defining characteristics of a binomial distribution 1 dichotomous outcomes (weights are on or off) 2 fixed number of trials (number of weights in the model doesn't change) 3 probability of success is fixed & independent for each trial.
$endgroup$
– Sycorax
1 hour ago
add a comment |
3
$begingroup$
I do not think that most implementations of dropout work by saying: If there are 100 neurons, and the probability is 0.05, I have to disable exactly 5 neurons chosen at random. Instead each neuron is disabled with a probability of 0.05, independently of what happens with the rest. Hence, the cases where all or no neurons are disabled, while unlikely, are possible.
$endgroup$
– Daniel López
13 hours ago
1
$begingroup$
@DanielLópez: I think both you and Djib2011 (+1 both) are "factually correct" on this. The statement is clearly oversimplifying things. You also need to take into account that most the networks that this paper is concerned with, have thousands of neurons so certain it is kind of accepted that no layer will be totally switched off.
$endgroup$
– usεr11852
13 hours ago
2
$begingroup$
Agree, but I believe the above example is transmitting the idea that exactly $n cdot textprob$ units are disabled with dropout, where $textprob$ is the dropout probability. And this is not how dropout works.
$endgroup$
– Daniel López
13 hours ago
$begingroup$
Well... LLN is our friend. :)
$endgroup$
– usεr11852
13 hours ago
$begingroup$
The flaw with the reasoning presented here is that dropout sets weights to 0 with some fixed probability independently. This implies that the number of zero weights at each step has a binomial distribution, because dropout has the three defining characteristics of a binomial distribution 1 dichotomous outcomes (weights are on or off) 2 fixed number of trials (number of weights in the model doesn't change) 3 probability of success is fixed & independent for each trial.
$endgroup$
– Sycorax
1 hour ago
3
3
$begingroup$
I do not think that most implementations of dropout work by saying: If there are 100 neurons, and the probability is 0.05, I have to disable exactly 5 neurons chosen at random. Instead each neuron is disabled with a probability of 0.05, independently of what happens with the rest. Hence, the cases where all or no neurons are disabled, while unlikely, are possible.
$endgroup$
– Daniel López
13 hours ago
$begingroup$
I do not think that most implementations of dropout work by saying: If there are 100 neurons, and the probability is 0.05, I have to disable exactly 5 neurons chosen at random. Instead each neuron is disabled with a probability of 0.05, independently of what happens with the rest. Hence, the cases where all or no neurons are disabled, while unlikely, are possible.
$endgroup$
– Daniel López
13 hours ago
1
1
$begingroup$
@DanielLópez: I think both you and Djib2011 (+1 both) are "factually correct" on this. The statement is clearly oversimplifying things. You also need to take into account that most the networks that this paper is concerned with, have thousands of neurons so certain it is kind of accepted that no layer will be totally switched off.
$endgroup$
– usεr11852
13 hours ago
$begingroup$
@DanielLópez: I think both you and Djib2011 (+1 both) are "factually correct" on this. The statement is clearly oversimplifying things. You also need to take into account that most the networks that this paper is concerned with, have thousands of neurons so certain it is kind of accepted that no layer will be totally switched off.
$endgroup$
– usεr11852
13 hours ago
2
2
$begingroup$
Agree, but I believe the above example is transmitting the idea that exactly $n cdot textprob$ units are disabled with dropout, where $textprob$ is the dropout probability. And this is not how dropout works.
$endgroup$
– Daniel López
13 hours ago
$begingroup$
Agree, but I believe the above example is transmitting the idea that exactly $n cdot textprob$ units are disabled with dropout, where $textprob$ is the dropout probability. And this is not how dropout works.
$endgroup$
– Daniel López
13 hours ago
$begingroup$
Well... LLN is our friend. :)
$endgroup$
– usεr11852
13 hours ago
$begingroup$
Well... LLN is our friend. :)
$endgroup$
– usεr11852
13 hours ago
$begingroup$
The flaw with the reasoning presented here is that dropout sets weights to 0 with some fixed probability independently. This implies that the number of zero weights at each step has a binomial distribution, because dropout has the three defining characteristics of a binomial distribution 1 dichotomous outcomes (weights are on or off) 2 fixed number of trials (number of weights in the model doesn't change) 3 probability of success is fixed & independent for each trial.
$endgroup$
– Sycorax
1 hour ago
$begingroup$
The flaw with the reasoning presented here is that dropout sets weights to 0 with some fixed probability independently. This implies that the number of zero weights at each step has a binomial distribution, because dropout has the three defining characteristics of a binomial distribution 1 dichotomous outcomes (weights are on or off) 2 fixed number of trials (number of weights in the model doesn't change) 3 probability of success is fixed & independent for each trial.
$endgroup$
– Sycorax
1 hour ago
add a comment |
ashirwad is a new contributor. Be nice, and check out our Code of Conduct.
ashirwad is a new contributor. Be nice, and check out our Code of Conduct.
ashirwad is a new contributor. Be nice, and check out our Code of Conduct.
ashirwad is a new contributor. Be nice, and check out our Code of Conduct.
Thanks for contributing an answer to Cross Validated!
- Please be sure to answer the question. Provide details and share your research!
But avoid …
- Asking for help, clarification, or responding to other answers.
- Making statements based on opinion; back them up with references or personal experience.
Use MathJax to format equations. MathJax reference.
To learn more, see our tips on writing great answers.
Sign up or log in
StackExchange.ready(function ()
StackExchange.helpers.onClickDraftSave('#login-link');
);
Sign up using Google
Sign up using Facebook
Sign up using Email and Password
Post as a guest
Required, but never shown
StackExchange.ready(
function ()
StackExchange.openid.initPostLogin('.new-post-login', 'https%3a%2f%2fstats.stackexchange.com%2fquestions%2f398114%2fcreating-thinned-models-in-during-dropout-process%23new-answer', 'question_page');
);
Post as a guest
Required, but never shown
Sign up or log in
StackExchange.ready(function ()
StackExchange.helpers.onClickDraftSave('#login-link');
);
Sign up using Google
Sign up using Facebook
Sign up using Email and Password
Post as a guest
Required, but never shown
Sign up or log in
StackExchange.ready(function ()
StackExchange.helpers.onClickDraftSave('#login-link');
);
Sign up using Google
Sign up using Facebook
Sign up using Email and Password
Post as a guest
Required, but never shown
Sign up or log in
StackExchange.ready(function ()
StackExchange.helpers.onClickDraftSave('#login-link');
);
Sign up using Google
Sign up using Facebook
Sign up using Email and Password
Sign up using Google
Sign up using Facebook
Sign up using Email and Password
Post as a guest
Required, but never shown
Required, but never shown
Required, but never shown
Required, but never shown
Required, but never shown
Required, but never shown
Required, but never shown
Required, but never shown
Required, but never shown
3N ehDN